How the brain can learn different melodies
Johanni Brea, Walter Senn and Jean-Pascal Pfister from the Institute of Physiology at the University of Bern propose a mathematical model in the "Journal of Neuroscience" that extends the so-called Hebb's learning rule for programming nerve connections in the brain.
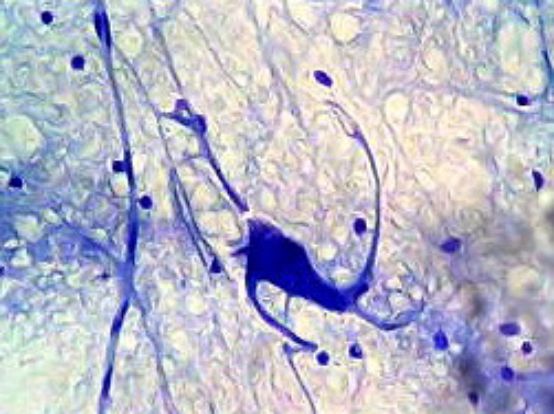
The starting point of the Bernese researchers' theory is a distinction between foreground and background neurons. The foreground neurons represent the activation patterns that are predetermined by a sequence, in the case of a melody by auditory neuronal foreground activity. While the sequence takes place in the foreground neurons, background neurons are also active in an initially random order. These learn to support the sequence of foreground activity.
According to Hebb's rule, connections between neurons activated in immediate succession are strengthened ("fire together, wire together"). However, the synaptic connections to the background neurons must not be adapted according to this rule, as otherwise the initially random and possibly incorrect sequences will "burn in" to the background neurons.
"According to our model, the synaptic changes are modulated by a signal that estimates the effect of the background activity on the foreground activity," explains last author Jean-Pascal Pfister: "If the current background activity has a supportive effect, the original Hebb learning rule is applied - otherwise the sign of the learning rule is reversed and the connection of sequentially activated neurons is weakened.
"In the case of the melody, this means that the background activity that would trigger an early or incorrect continuation of the melody is suppressed within the pause," adds Pfister.
The Bern researchers' model makes experimentally directly testable predictions. How exactly higher-level signals adapt the synaptic connections can be determined experimentally, Pfister continues.
Original article:
Johanni Brea, Walter Senn and Jean-Pascal Pfister: Matching storage and recall in sequence learning with spiking neural networks. The Journal of Neuroscience, June, 5 2013, 33(23): 9565-9575; doi: 10.1523/JNEUROSCI.4098-12.2013